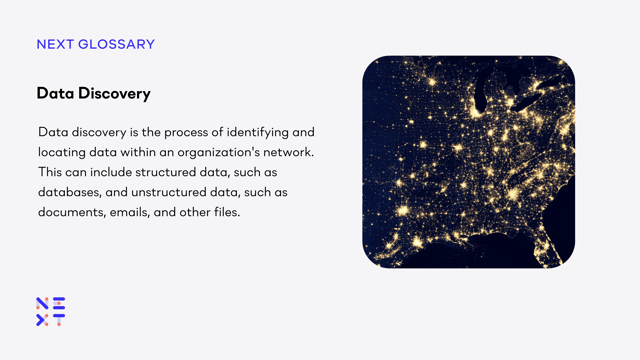
Data discovery refers to the process of identifying and locating sensitive data within an organization's network, systems, and storage repositories. It involves scanning, analyzing, and categorizing data to understand its nature, location, and level of sensitivity.
Data discovery has long been considered a crucial component of DLP and insider risk management strategies as it helps organizations gain visibility into their data landscape and implement appropriate security controls.
Let's explore the pros and cons of data discovery:
Pros of Data Discovery for DLP and Insider Risk Management:
- Enhanced Data Visibility:
Data discovery provides organizations with comprehensive visibility into their data landscape. It helps identify and locate sensitive data assets, including personally identifiable information (PII), financial records, intellectual property, or confidential business data. This enhanced visibility enables organizations to effectively manage and protect their data assets.
- Improved Data Classification:
Data discovery facilitates the classification of data based on its sensitivity and regulatory requirements. By scanning and analyzing data, organizations can automatically or manually assign appropriate classifications and labels to the data. This allows for better understanding and control of data access, usage, and protection measures.
- Risk Mitigation:
Data discovery enables organizations to identify data exposures and vulnerabilities. By scanning data repositories, networks, and systems, organizations can detect potential risks such as data leaks, unauthorized access, or policy violations. This early identification helps organizations implement proactive security measures to mitigate risks and prevent data breaches.
- Regulatory Compliance:
Data discovery supports organizations in meeting regulatory compliance requirements. It helps identify data that falls under specific regulations, such as the General Data Protection Regulation (GDPR), Health Insurance Portability and Accountability Act (HIPAA), or Payment Card Industry Data Security Standard (PCI DSS). By understanding where sensitive data resides, organizations can ensure compliance, implement appropriate security controls, and manage data retention and deletion effectively.
- Insider Threat Detection:
Data discovery assists in detecting insider threats by monitoring and analyzing data access and usage patterns. It enables organizations to identify suspicious activities, such as excessive access to sensitive data, unusual data transfers, or unauthorized file sharing. By correlating this information with user behavior analytics, organizations can identify potential insider risks and take appropriate actions.
- Data Lifecycle Management:
Data discovery helps organizations gain insights into the entire data lifecycle. By understanding where data is stored, how it is accessed, and who has access to it, organizations can implement effective data management practices. This includes data retention policies, data backup and recovery strategies, and data disposal processes.
Cons of Data Discovery for DLP and Insider Risk Management:
- False Positives and False Negatives:
Data discovery techniques may generate false positives or false negatives during the scanning and analysis process. False positives occur when data is incorrectly identified as sensitive, leading to unnecessary alerts or actions. False negatives occur when sensitive data goes undetected, potentially leaving it exposed to risks. Organizations need to fine-tune data discovery tools and algorithms to minimize these errors.
- Scalability and Performance Impact:
Data discovery can be resource-intensive, particularly in large and complex data environments. Scanning and analyzing extensive data repositories can impact system performance and require significant processing power. Organizations must carefully plan and implement data discovery processes to minimize disruption and ensure scalability.
- Privacy and Data Protection Concerns:
Data discovery involves scanning and analyzing data, which raises privacy and data protection concerns. Organizations must ensure compliance with relevant privacy regulations and adopt appropriate security measures to protect the confidentiality and integrity of the data being analyzed. Privacy considerations should be given to personal data and sensitive business information.
- Data Source Compatibility:
Data discovery tools may face challenges when scanning and analyzing data from various sources and formats. Compatibility issues may arise when dealing with legacy systems, proprietary databases, or unstructured data formats. Organizations should ensure that their data discovery solutions support a wide range of data sources and formats to maximize coverage and effectiveness.
- Maintenance and Updates:
Data discovery requires ongoing maintenance and updates to remain effective. As data repositories and systems change over time, organizations need to regularly update their data discovery processes to ensure accurate and up-to-date results. This includes adding new data sources, modifying scanning rules, and adjusting classification algorithms as the data landscape evolves.
- Complexity and Deployment Challenges:
Implementing data discovery solutions can be complex, requiring expertise and resources. Organizations need to carefully plan the deployment, configuration, and integration of data discovery tools within their existing IT infrastructure. Adequate training and support for administrators and users are essential to ensure successful implementation and utilization.
Relevance to Insider Risk and Data Protection
Data discovery is a vital component of DLP and insider risk management strategies, providing organizations with enhanced data visibility, improved risk mitigation, regulatory compliance, and insider threat detection. While data discovery offers significant benefits, organizations must be mindful of the potential challenges, such as false positives/negatives, scalability, privacy concerns, data source compatibility, maintenance, and deployment complexities.
By understanding these pros and cons, organizations can make informed decisions when implementing data discovery solutions to strengthen their data protection and risk management efforts.