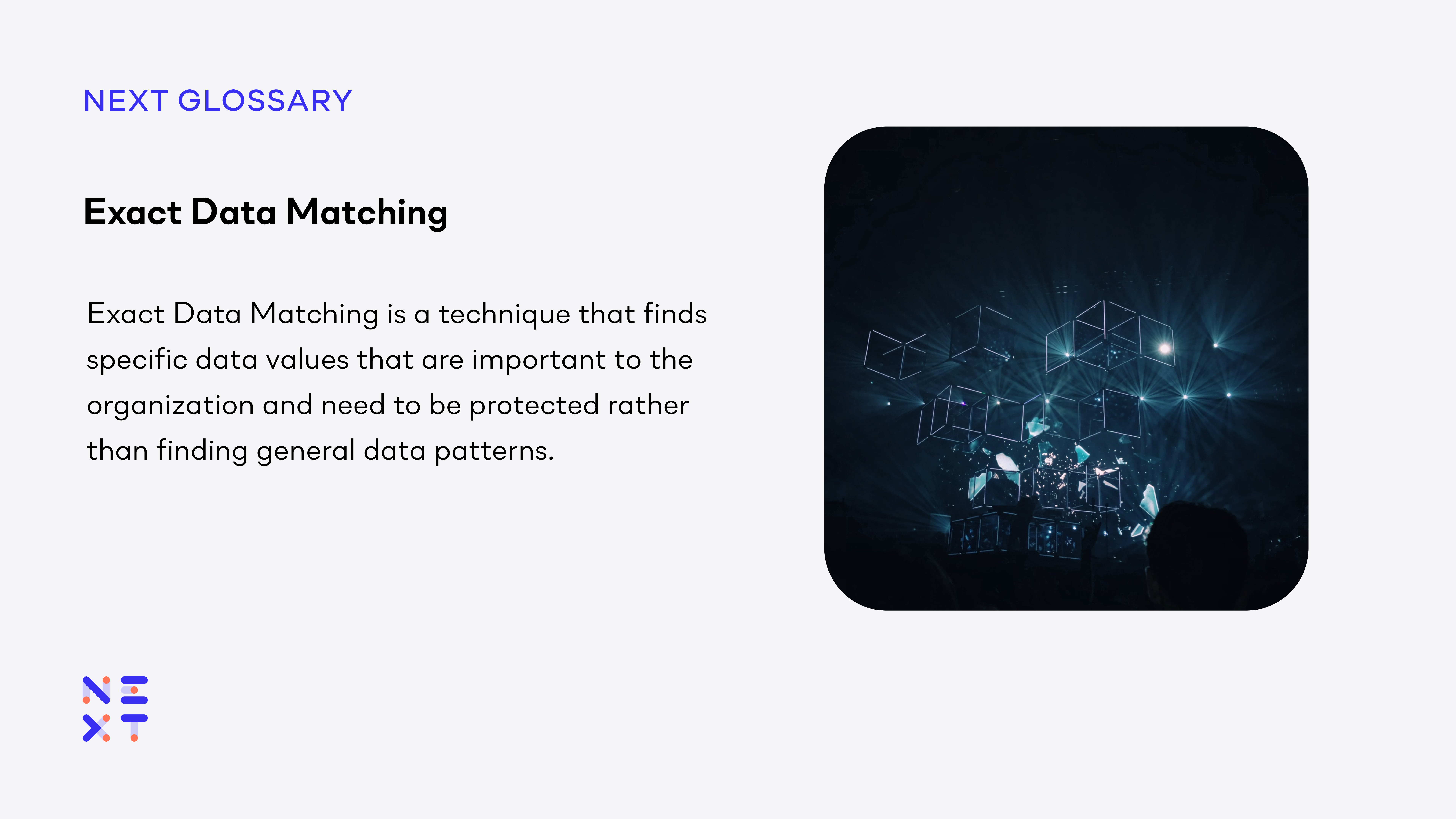
Data matching or exact data matching (EDM) refers to the process of comparing and correlating data elements or patterns to identify matches or similarities between different data sources. It involves analyzing data to identify instances where sensitive or confidential information is present, enabling organizations to detect policy violations, data leaks, or insider threats.
Let's explore the pros and cons of data matching:
Pros of Data Matching for DLP and Insider Risk Management:
- Detection of Sensitive Data:
Data matching helps organizations identify sensitive data by comparing data elements or patterns against predefined criteria. It enables the detection of sensitive information such as personally identifiable information (PII), financial data, intellectual property, or confidential business data. By matching data across various sources, organizations can effectively locate and protect sensitive data within their environment.
- Policy Enforcement:
Data matching plays a crucial role in enforcing data protection policies. It allows organizations to define specific rules or patterns that indicate policy violations, such as unauthorized sharing of sensitive information or excessive data access. By comparing data against these predefined rules, organizations can identify and address policy violations promptly.
- Insider Threat Detection:
Data matching assists in insider threat detection by correlating data elements associated with user activities. By comparing data patterns, access logs, or transaction records, organizations can identify anomalous behavior, such as unusual data transfers, unauthorized access, or suspicious data access patterns. Data matching helps detect potential insider risks and supports proactive mitigation measures.
- Data Leakage Prevention:
Data matching helps prevent data leakage by identifying instances where sensitive data is being transmitted or shared outside the organization. By comparing data against known patterns or predefined rules, organizations can detect unauthorized data transfers, unauthorized sharing of confidential information, or attempts to bypass security controls. This enables proactive intervention to prevent data leaks or unauthorized disclosures.
- Compliance with Regulations:
Data matching supports organizations in complying with data protection regulations and industry standards. By comparing data against specific criteria defined by regulatory requirements, organizations can ensure compliance with regulations such as the General Data Protection Regulation (GDPR), Health Insurance Portability and Accountability Act (HIPAA), or Payment Card Industry Data Security Standard (PCI DSS). Data matching aids in identifying and protecting data that falls under regulatory obligations.
Cons of Data Matching for DLP and Insider Risk Management:
- False Positives and False Negatives:
Data matching techniques may generate false positives or false negatives during the matching process. False positives occur when data is incorrectly identified as sensitive or a policy violation, leading to unnecessary alerts or actions. False negatives occur when sensitive data or policy violations go undetected, potentially leaving organizations exposed to risks. Fine-tuning the matching algorithms and rules is crucial to minimize these errors.
- Complexity of Matching Rules:
Developing and maintaining matching rules can be complex. Defining accurate and comprehensive rules that cover all sensitive data patterns or policy violations requires careful analysis and continuous updates. Organizations must invest time and resources in creating effective matching rules to minimize false positives and ensure accurate detection.
- Scalability and Performance Impact:
Data matching can be resource-intensive, particularly when dealing with large volumes of data or complex matching rules. Matching data across multiple sources and analyzing patterns can impact system performance and require significant processing power. Organizations should consider scalability and performance considerations when implementing data matching solutions.
- Privacy and Data Protection Concerns:
Data matching involves analyzing and comparing data, which raises privacy and data protection concerns. Organizations must ensure compliance with relevant privacy regulations and adopt appropriate security measures to protect the confidentiality and integrity of the data being matched. Privacy considerations should be given to personal data and sensitive business information.
- Maintenance and Updates:
Data matching rules and algorithms require ongoing maintenance and updates to remain effective. As data patterns evolve, new threats emerge, or regulatory requirements change, organizations need to regularly update their matching rules and algorithms to ensure accurate and up-to-date detection. This requires monitoring industry trends, staying informed about new data patterns, and aligning the matching rules with evolving risk landscapes.
- Data Source Compatibility:
Data matching may face challenges when dealing with diverse data sources and formats. Compatibility issues may arise when matching data from different systems, databases, or file formats. Organizations should ensure that their data matching solutions support a wide range of data sources and formats to maximize coverage and effectiveness.
Data Matching: Relevance to Insider Risk and Data Protection
Data matching is a valuable technique for DLP and insider risk management, enabling organizations to detect sensitive data, enforce policies, prevent data leakage, and identify insider threats. While data matching offers significant benefits, organizations should be mindful of the potential challenges, such as false positives/negatives, complexity of matching rules, scalability considerations, privacy concerns, maintenance requirements, and data source compatibility.
By considering these pros and cons of data matching, organizations can make informed decisions when implementing data matching solutions to enhance their data protection and risk management efforts.